AI Benchmarks Just Dropped! Nvidia’s New Chips Crush the Competition
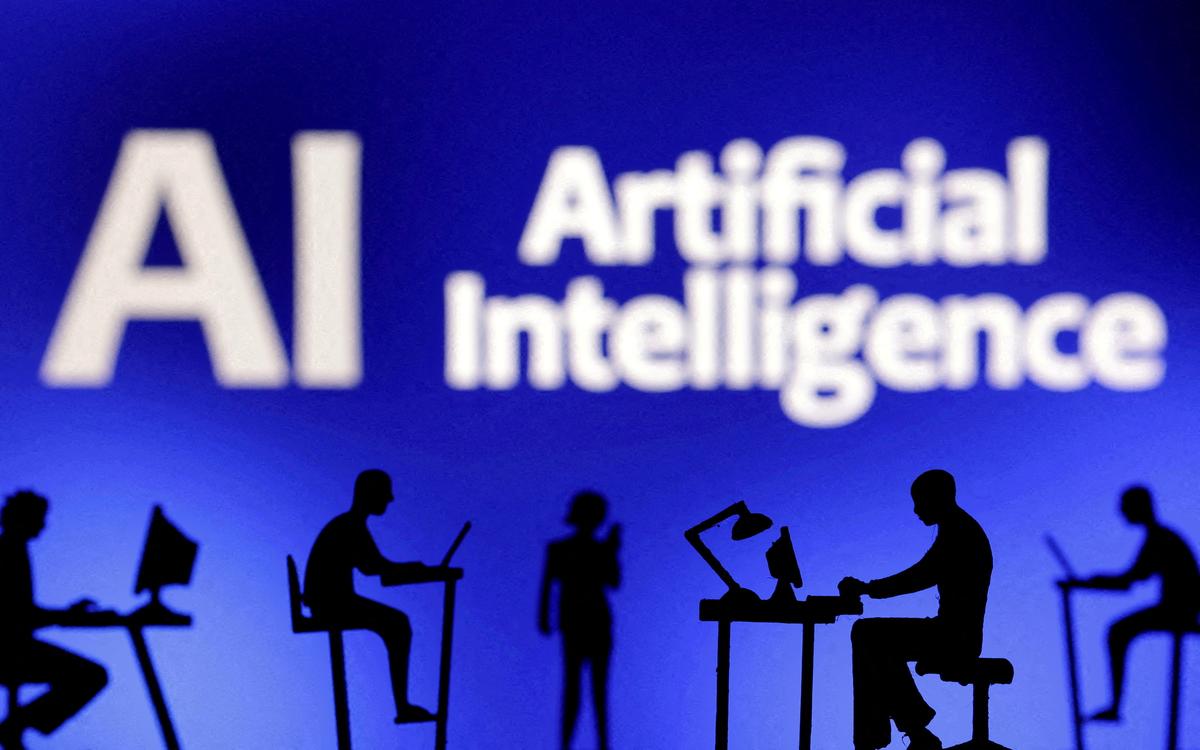
New AI Benchmarks Set to Test the Speed of AI Applications
MLCommons Introduces Two New AI Performance Benchmarks
Artificial intelligence is evolving at a rapid pace, and so is the hardware that powers it. In response to this, MLCommons, a leading AI benchmarking group, has unveiled two new AI benchmarks designed to test how efficiently hardware and software can run AI applications. These benchmarks are critical as companies race to develop faster and more capable AI-powered systems.
Why These AI Benchmarks Matter
Since the launch of OpenAI’s ChatGPT, AI has seen widespread adoption across industries. This surge in AI applications has led to an increased demand for powerful hardware that can handle complex computations at high speeds. Tech giants like Nvidia, Dell, and Meta are constantly working to improve AI performance, and these benchmarks will help gauge just how efficient their hardware and software solutions really are.
Benchmark 1: Testing Meta’s Llama 3.1 AI Model
The first new benchmark is built around Meta’s Llama 3.1 AI model, which boasts an impressive 405 billion parameters. This benchmark is specifically designed to evaluate systems on tasks such as:
- General question answering
- Mathematical problem-solving
- Code generation
With AI models becoming increasingly complex, this test will determine how quickly and accurately AI-powered systems can process large queries and synthesize data from multiple sources.
Nvidia Takes the Lead in AI Benchmarking
Nvidia, a dominant player in the AI hardware industry, submitted multiple chips for this benchmark. System builders like Dell Technologies also participated. According to MLCommons' data, Advanced Micro Devices (AMD) did not submit any entries for the 405-billion-parameter benchmark.
Nvidia reported that its latest AI server, Grace Blackwell, outperformed its previous generation of AI servers by 2.8 to 3.4 times in this benchmark. Even when testing with just eight GPUs (as a direct comparison to the previous model), Grace Blackwell demonstrated significant speed improvements. The company attributed this performance boost to its work in optimizing chip connectivity within its servers.
Benchmark 2: Simulating Real-World AI Application Performance
The second benchmark, also based on an open-source AI model developed by Meta, is designed to replicate real-world performance scenarios. It aims to set realistic response time expectations for consumer AI applications, such as:
- AI chatbots
- Search engines
- Virtual assistants
One of the primary goals of this benchmark is to reduce AI response times, bringing them closer to instantaneous replies. This is particularly important for AI-powered applications where users expect seamless and natural interactions.
The Race for AI Speed and Efficiency
As AI applications become more complex, the need for high-speed, efficient hardware grows. These new MLPerf benchmarks from MLCommons provide a standardized way to measure how different AI systems perform under real-world conditions. With companies like Nvidia leading the charge, the competition to build the fastest AI hardware is heating up.
What’s Next for AI Hardware?
With the introduction of these benchmarks, tech companies now have a new standard for measuring and improving their AI performance. As AI technology continues to advance, these tests will play a crucial role in pushing the boundaries of what AI systems can achieve.